Subscribe to join thousands of other ecommerce experts
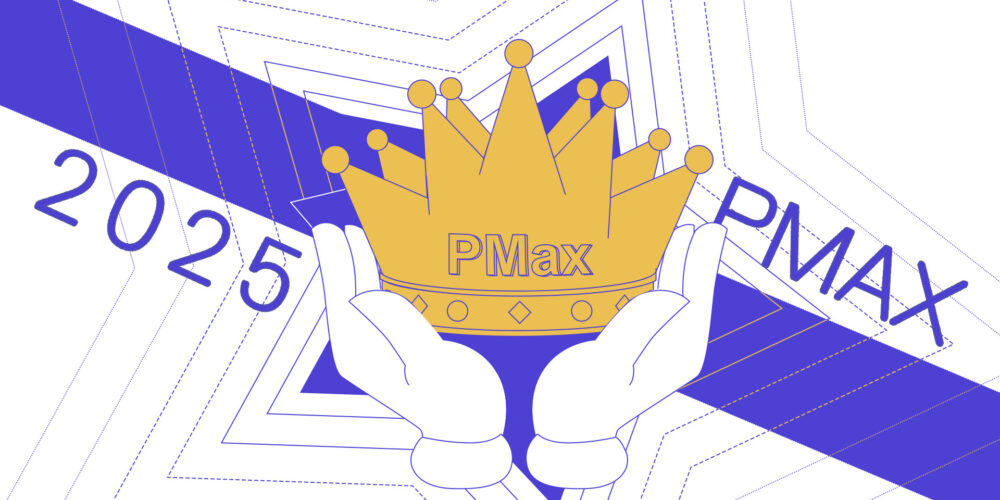
What? Another “How to PMax” article? Yes, but this one’s the most comprehensive you’ll read yet!
In 2024 alone, we’ve set up over 700 campaigns for our clients. Refining PMax optimization techniques to drive profitable ecommerce growth.
This article isn’t just a guide; it’s a look inside how to steer the AI for maximum impact. So let’s break it down:
Table of Contents
PMax optimization: Where we’re coming from
Google PMax has been around for a while now. I don’t want to sound nostalgic, but back in the “good old days” of manual bidding on product and listing groups, we had tools for precise campaign adjustments. Now, we’re left with setting a tROAS and budget at the campaign level—quite the shift from maximum control to just a few levers.
This evolution makes campaign management easier on the surface but introduces challenges for those who don’t focus on PMax optimization. Without a strategic approach, Performance Max is effectively a Black Box, chasing quick wins at the expense of long-term goals.
Maximum control → Max number of campaigns?
It seems logical to think that more campaigns equal more control. But as we’ve seen with Performance Max over the years, spreading conversion data too thin can actually hurt performance. Our analysis shows that lower data density per campaign often results in worse tROAS outcomes.
Take a look at the underperforming sections of the bars above: campaigns with insufficient data struggle to hit their targets. You’ll basically hand all your control over to PMax and have no control over which products are served, nor where and how aggressively. Do you really want this?
The key takeaway? When it comes to PMax optimization, success isn’t about creating more campaigns but building smarter structures that balance data density with segmentation.
4 approaches for PMax optimization
Honestly, navigating Performance Max can feel a bit like exploring a maze without a map—there’s potential around every corner, but it’s easy to get lost.
The key to effectively optimizing Performance Max lies in how you structure your campaigns. A solid setup can steer the algorithm toward profitable results, while a misstep can leave performance on the table.
We’ve identified four common approaches to structuring PMax campaigns, ranging from entry-level setups to advanced, data-driven strategies.
Whether you’re just starting out or looking to refine your current setup, understanding these methods can help you unlock the full potential of PMax:
Worst case: 1 Campaign = Black Box
This is Google’s favorite starting point for PMax optimization: one full-funnel campaign where you set a budget, add a tROAS, and sit back. It’s simple and gets you running, but here’s the catch—it doesn’t take your unique business needs into account.
Google optimizes auction by auction using only the data it can see. This often means a handful of overachieving products dominate the ad spend and revenue. If one of those “heroes” goes out of stock, your performance might nosedive.
When this approach works for you:
- You’re new to PMax optimization and want to gather first learnings.
- Your product catalog is small and straightforward.
If you have large catalogs, and/or focus on long-term growth, this is just a stepping stone.
Bad case: 1-Dimensional Segmentation
This setup is where PMax optimization can go off the rails. Segmenting products by a single attribute, like margins or historical performance, sounds smart but often creates more problems than it solves.
We understand under a 1-dimensional segmentation two different scenarios:
- Campaigns that are built based on 1 attribute in the feed (eg high-mid-low margin)
- Campaigns that just use 1 item ID’s performance snapshots to cluster products (you’ll later see a 2-d matrix which we still consider as 1d segmentation 🤯)
Let’s keep in mind, that we’d like to steer the AI in a profitable way and you might wonder why a campaign setup of putting products with similar margins together, has just little to do with profitability?
#1: Campaigns built on 1 attribute in the feed
With most of the retailers we’ve analysed (eg by adjusting the pixel with conversions with cart data), we noticed that approx. 50% of the products that have been clicked, were either not purchased at all or were bought alongside some other products.
Meaning, the margin of clicked products is in most of the cases something completely different than the actual basket margin. If you’re now pushing products with high margins (which are – let’s be honest – often not THE most attractive products in your assortment), it will simply lead to more clicks on these products.
What happens later in the shop is totally out of the hand of a PPC campaign.
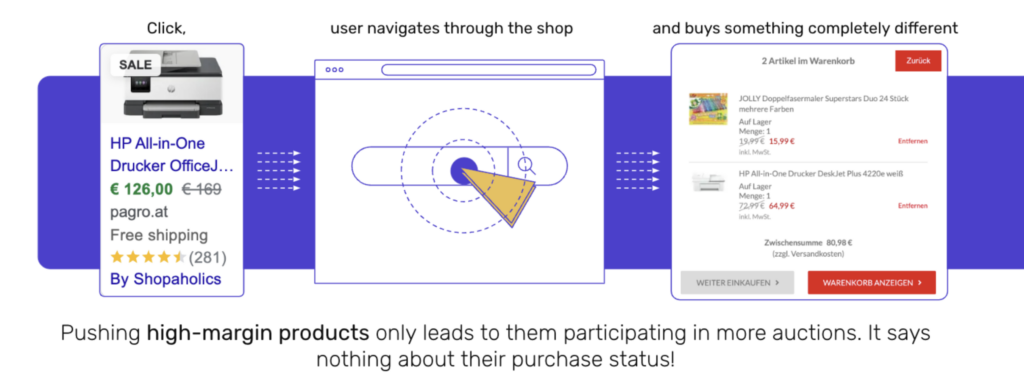
And here’s yet another issue: just because you cluster products based on margin doesn’t mean those products are in demand, relevant, or in season. Ecommerce success isn’t driven by a single metric—it’s influenced by consumer behavior, market trends, seasonality, and inventory dynamics.
A 1-dimensional view neglects these complexities, leading to campaigns that fail to resonate with shoppers.
#2: Campaigns with 1 item ID snapshot:
The other 1-d segmentation possibilities are frameworks where you cluster products based on their volume and efficiency, often by scripts.
You define a threshold for which you’d think that the product is high/low in efficiency and high/low in volume and end up with 4 different groups.
This leads to 80-90% of products with little data ending up in campaign #1, 1-3% in campaign #4, which then receive a disproportionately high/low budget and leave the long-tail products to their fate.
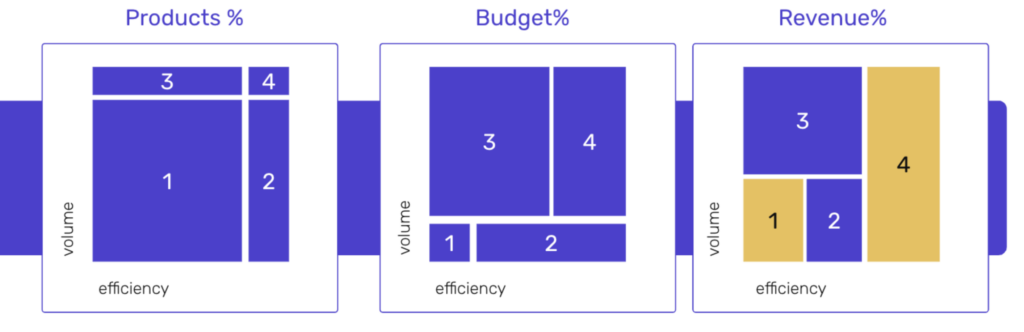
When this approach works for you:
- You’re focusing on short-term ROAS over strategic growth.
- Your PMax optimization process lacks access to more nuanced data.
If that sounds limiting, it’s because it is. So let’s make it smarter:
Ok case: PMax scoring based on Business Data
Here, PMax optimization starts getting smarter. But why do we even need business data in the campaign structure? Well, there are simply some metrics that are more important for customers, Heads of Ecommerce or CFOs, or Google Ads than others. This means it’s our job as PPC managers to steer the AI in the right direction.
Step 1:
So, think about the nature of your business and add relevant data into your Data Feed using Custom Labels:
- What USPs do your customers value?
- Which metrics are brought up by your colleagues in controlling?
- What does Google Ads say about previous performance?
Think gross margin, stock availability, or seasonal demand—all factors that matter in ecommerce but aren’t visible to the algorithm.
Step 2:
Next, you’ll need the data in a (Google) spreadsheet, to define the weighting. Each of your products receive a numeric score and you may then send this information by to Google in order to create a custom label in the Merchant Center. You can use these custom labels when creating PMax campaigns and their listing groups in Google Ads.
If you run this script once per day and add some fallback logic to it, this approach can provide a temporary boost by feeding the AI more business-relevant information at the product level.
However, let’s not sugarcoat it: refreshing data for thousands of products daily is a grueling task that’s prone to errors. It’s a manual, time-intensive process that could break under the weight of scaling.
At some point, you’ll inevitably hit a wall—not just with spreadsheet limits but with the effort required to keep the data accurate and up-to-date. Expanding beyond the constraints of 4 custom labels or incorporating additional metrics becomes an uphill battle.
When this approach works for you:
- You have a team familiar with data tools and scripting.
- You’ve started integrating business metrics into your PMax campaigns.
This approach might work as a stopgap, but it’s far from a robust or sustainable solution for businesses with larger catalogs or long-term goals.
Best Case: Multi-Dimensional Segmentation
Welcome to the pinnacle of PMax optimization.
Multi-dimensional segmentation takes product categorization to the next level by integrating predictive algorithms, historical data, and strategic business insights. This approach doesn’t just improve campaign performance—it aligns your campaigns with your overarching business goals.
Step 1: Establish a Base Score
The foundation of this approach is a Base Score, which ranks products from low to high based on their past and predicted future performance. For SKUs with little or no historical data, a fallback model can leverage product neighborhoods (similar products) to estimate potential. To ensure new products don’t get left behind, incorporate a boost for new arrivals. Customization at every stage is crucial to adapt the scoring to your business needs.
For example, in some accounts, the number of conversions might take priority over the conversion value—and your scoring system should reflect this flexibility.
Step 2: Add strategic data
Once you have a base score, layer on strategic data that reflects your industry and goals. The first question to ask is: What data makes sense for my business as a [retailer type] with [specific strategic goals]?
Here’s how different goals can guide the data you prioritize:
- Revenue growth: Focus on price competitiveness, stock index, and seasonality.
- Profit growth: Incorporate gross margin in conversion value, actual shipping costs, and products leading to high CLVs.
- Cost reductions: Use data like return rates and fulfillment costs to refine scoring.
If you’re in fashion, seasonality might dominate your KPIs, while an electronics retailer might weigh margins more heavily. Multi-brand shops could prioritize product margins, unlike single-brand retailers.
Step 3: Address data scarcity with strategic scoring
Now combine the Base Score with strategic data to address challenges like data scarcity and guide PMax’s focus. For instance, if your Non-Shopping spend exceeds 30%, consider scoring not just products but also landing pages.
With this strategy, high-scoring products or pages don’t necessarily have the most traffic or efficiency, but they excel in both past performance and strategic relevance.
Data Science: The secret ingredient
Executing this strategy requires advanced data science capabilities to build and refine the scoring model. So, are you ready to take this on alone?
That’s where we can help you out quite a bit. 😃 With our tools and expertise, we can simplify the process and unlock PMax’s full potential for your business.
When this approach works for you:
- You’re ready to invest in advanced tools or partner with experts who specialize in PMax optimization.
- You want to unlock the full potential of your campaigns through data-driven strategies.
PMax optimization: What’s Next?
PMax optimization isn’t just about setting up campaigns—it’s about constantly improving them. The most successful advertisers focus on feeding PMax with superior data, strategically guiding the algorithm, and refining their approach over time.
At smec, we specialize in turning PMax into a growth engine. From multi-dimensional segmentation to predictive budget allocation, we help ecommerce businesses unlock new opportunities and achieve sustainable success.
Ready to master PMax?
Don’t let your campaigns settle for average. Schedule a call with our experts today and discover how smarter strategies and data-driven insights can drive your business forward.
Let’s transform your challenges into growth opportunities.