Subscribe to join thousands of other ecommerce experts
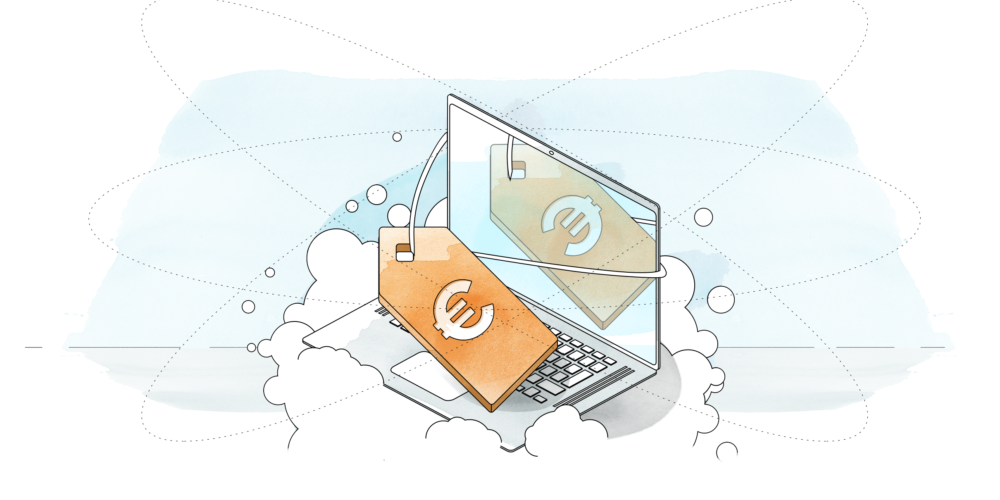
Table of Contents
Retail pricing optimization in a nutshell
Pricing decisions are among the most important decisions in ecommerce – and in retail in general, as proficient pricing optimization directly leads to increased revenue and profit.
While this might not surprise you, throughout this blogpost, I will reveal some pricing-related insights that are not so clear at the very first glance. We will start with findings gained through smec’s rich experience in data-driven bidding automation, discuss price elasticity in general, and finally, highlight various optimization possibilities in price, promotion and catalog management.
How pricing can influence your KPIs
Working with Shopping Ads and hundreds of retailers, we have learned a lot about the particularities of ecommerce. Among others, we discovered that price and price-related features are strong predictors for the conversion rate of a product. The price of a product determines how it is positioned in and perceived by the market – online shoppers typically make decisions by weighing how much they are willing to pay against the actual price of a product they come across. In ecommerce settings, it is quite easy and common for online shoppers to compare alternatives and prices from various brands or shops.
Competitor prices are by far one of the most important factors in driving price sensitivity: research shows that the highest price sensitivity is located near competitor prices. Even slight changes to your price – in this particular price range – could affect demand for better or worse. Hence, it’s essential for retailers to consider competitor price information in their pricing decisions.
With the importance of pricing in mind, let’s talk about the economic perspective on the relationship between price and demand.
How to calculate demand and price elasticity
In general, the relationship between price and demand is not new and has been studied for centuries. It is described in economics as the price-demand function and allows us to express revenue or profit as a function of product price:
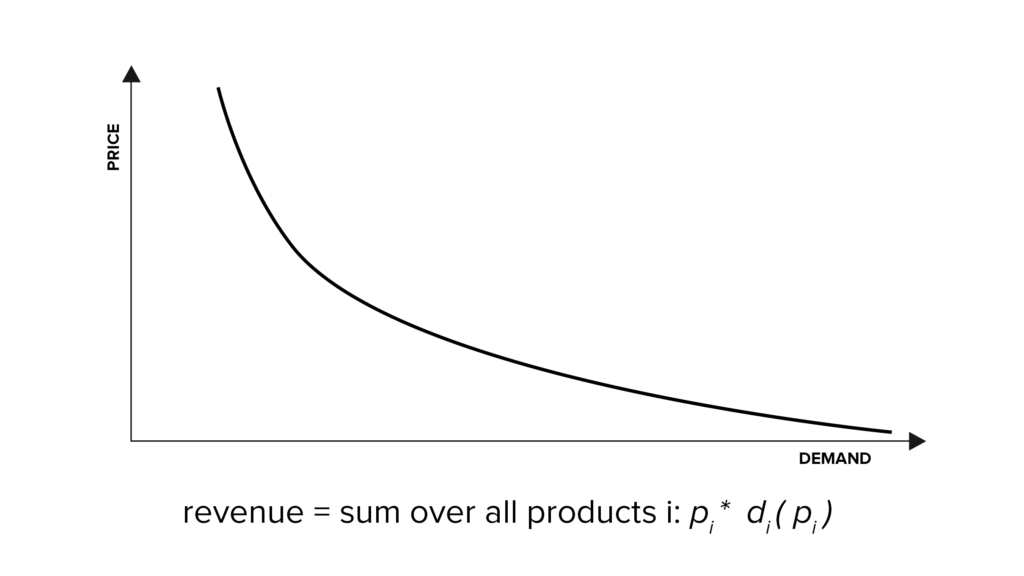
Another widely used term is price elasticity, which is a measure of how much demand changes as the price of the product changes. Mathematically, it is expressed as:
Price elasticity = % change in demand / % change in price
Clearly, we see that price and demand are closely coupled. Assuming that the price-demand function for a particular product is known, a profit-optimal price could be computed by solving the following optimization problem:
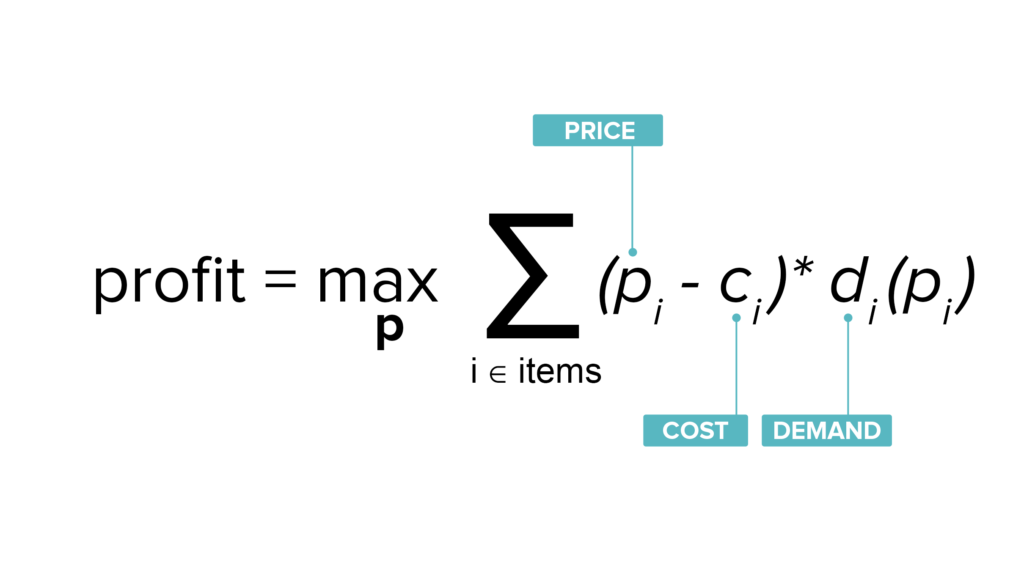
To put that into words, the profit function is systematically computed for all available price levels, taking into account the demand at that price, as well as the item’s costs. The price that results in the highest profit is then chosen and applied.
To illustrate this further, let’s assume the following scenario:
A certain product can be sold at the following prices: €19.99, €22.99, €25.99 or €27.99. The costs to the retailer for this particular product are €8.50 and the price-demand function is given. By solving the above optimization problem, the price €22.99 will be chosen as it results in the highest profit (about €304).
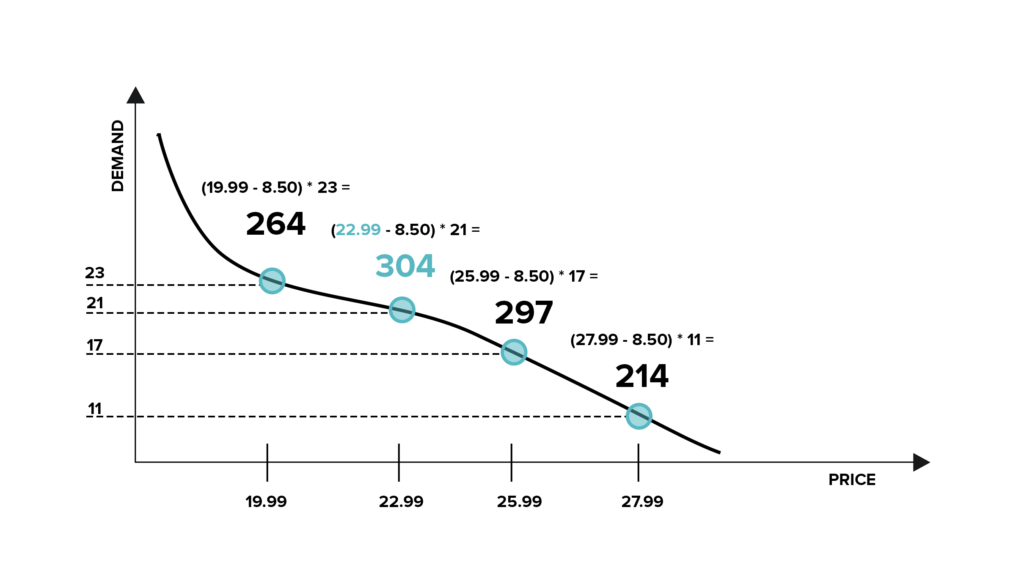
What to consider when calculating demand
Although the above looks promising, such an approach will hardly work out in the real world due to several constraints:
- The demand for a product is based on a complex interplay of various factors (in addition to price), such as substitutable products or competing offers.
- Demand is often dynamic and depends on seasonalities or short-term trends, which necessitates a regular re-estimation of the demand function.
- The demand-price relationship is typically unknown and can only be estimated.
How to conduct your demand forecast
By leveraging machine learning methods and historical sales data, it is possible to estimate advanced demand functions that incorporate various factors, ranging from product attributes (such as brand, price, and product type), offers of competitors and sales or promotional activities, to weather data and macroeconomic indices. Building such data models on a regular basis, allows you to perform ‘what-if simulations’ and to learn which impact price changes have.
When it comes to such AI-based techniques, the major prerequisite is always data: reliable and up-to-date data about your offers, acquired at a high velocity, is the foundation for any demand estimation. Don’t limit yourself to your own data: the data can be enriched with external sources. The more diverse the available data is, the more reliable the demand estimates will be.
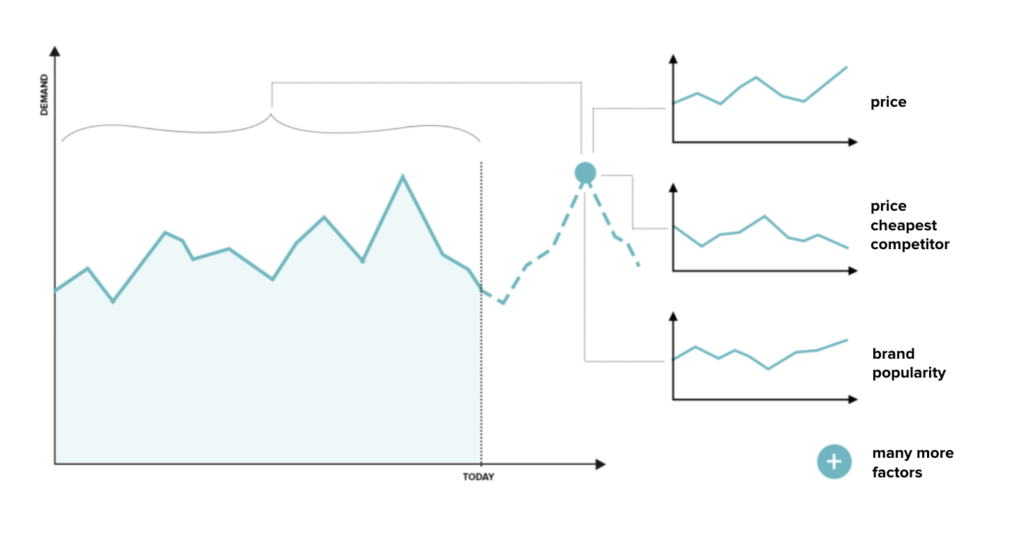
Data-driven repricing with competitor information
As mentioned earlier, prices and offers of your competitors are essential in guiding pricing and assortment decisions, and should be treated as valuable clues for demand predictions. If you are using a dynamic pricing tool, data about competitor prices can also be implemented as pricing strategies to, for example, make sure that prices for a defined product follow certain key competitors or undercut them by a certain percentage.
A further (more complex) step in automated price management would be to go beyond ‘rules’ and to implement AI-driven repricing. In general, however, all repricings (no matter whether rule- or AI-based) should be evaluated systematically and subject to rigorous A/B testing.
Use cases: Pricing management, catalog optimization and promotions
So far, I’ve only discussed demand forecasting from the perspective of price management. However, demand forecasting forms the basis for a variety of enterprise problems that I’ll briefly outline here:
Price management
As hinted at, intelligent price management deals with finding revenue- or profit-optimized prices in an algorithmic manner. It also helps to prevent overstock situations or running out of stock too early on. Also, introductory prices for new items can be set more easily. Technically, intelligent price management builds on AI-driven demand prediction methods that learn from historical sales data as well as from competitor prices, seasonalities, inventory constraints and cross-item elasticities (the change in demand of one product caused by the change in price of another).
However, price management can very well also be rule-based or manual, depending on data availability, automation capabilities and, of course, the retailer’s overall innovation strategy and business processes.
Catalog optimization and assortment insights
Catalog optimization refers to fine-tuning the set of offered items. Being able to predict product demands and having insights into cross-item elasticities and complementary product affinities enables retailers to estimate item profitabilities and, based on that revise their catalogs.
Promotion management
Questions about when to put which products on sale and for how much concern all retailers no matter which vertical. Automation can ease those pains. Promotion management solutions, which generate recommendations for sale periods and respective prices, again build on the foundation of demand predictions.
Intelligent pricing optimization is key
I hope this blog post helped to shed some light on the importance of informed pricing decisions. In order to support these decisions algorithmically, it is essential to collect historical pricing and sales data in a structured way. We also explored the value of external data sources, such as competitor price data. They can boost prediction accuracy and generate their own positive side effects, as we saw in the example of rule-based dynamic pricing.
The main message I want to convey is that if you have enough data available to study product demands, a major step towards the automated and intelligent management of prices, catalogs and promotions is already taken. If one of the topics introduced here really caught your attention, then be sure to keep an eye on smec’s software solution, which feeds the advertising algorithm with superior data inputs that allows you to fine-tune and sync your paid search campaigns with your goals for maximum impact.